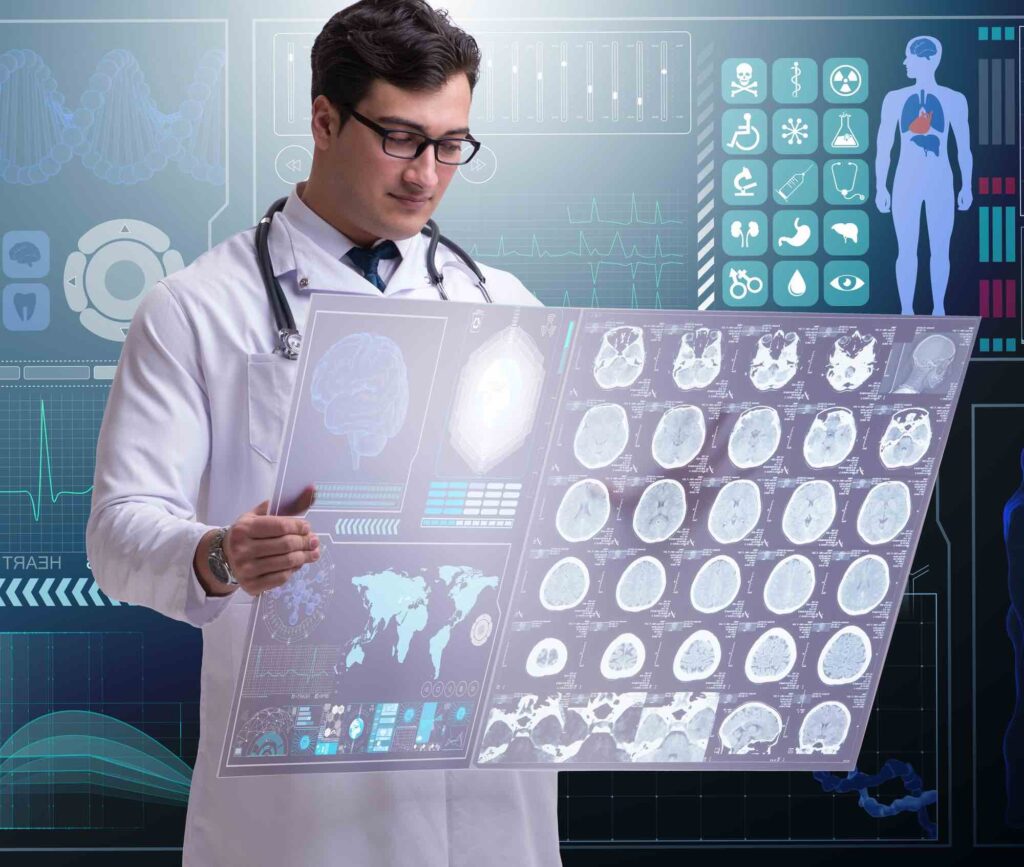
Are we ready to welcome our AI overlords into healthcare yet? Will we welcome a fully autonomous Dr. Crusher? We’re not likely ready to completely abandon our human healers, but there have been a variety of algorithms and machine learning applications that have been creeping their way into our clinical specialties for many years now. The first AI algorithm was approved by the FDA in 1995, in the beginning of 2023 there are 520. The specialty with the largest number? Radiology! Check out this article from HealthExec, it provides some more detail on the approval and advantages of AI in imaging.
Radiology, and similar specialties that utilize imaging, have long embraced computer systems to improve quality, productivity, and sharing. The complexity behind the scenes of these systems can be quite surprising. Once an image is captured it may still go through multiple systems just to be viewed, PAC Systems, VNA, Dicom viewers, the route is anything but direct. If we want to add another step in the process to have the image analyzed, we are faced with yet another connection.
In a previous blog post we pointed out the challenges that staff face when one of these systems aren’t communicating, these certainly impact our physicians as well. As we add more diagnostic solutions to aid our physicians in treating patients, we want to ensure that their time is actually spent doing what they are trained for. The average radiologist makes roughly $300,000 per year, if we assume that radiologist works 40 hours per week, that’s about $144 per hour.
What happens when the machines stop talking? Likely the radiologist calls the help desk… 5 minutes on hold ($12), they will take 3 minutes to explain the problem to the help desk person ($7.20), who will then transfer them to a specialist where they take another 5 minutes talking over the problem ($12). Helpdesk will then forward the issue to multiple analysts and integration team. At-least 2 different analysts getting pulled into diagnosing the issue. Typically, integration and system analysts make 90k-130k per year or on average $50 per hour. These 2 analysts will work on this issue for about 30min ($100) to diagnose the issue. $131.20 may not seem like a significant amount, but now that radiologist may not be reviewing images, may not be discussing treatments with patients, they may not be doing what they’ve been trained to do. If the radiologist is only 50% as productive without this solution, every hour of outage costs $132… That’s just for one Radiologist. And typically system issues affect all users. So once you multiply the outage costs for all radiologists and other team members it really adds up fast. There can also be significant downstream impacts to other services waiting on imaging as well that become harder to calculate.
Moving beyond the frustration of the healthcare teams, there can be a detrimental impact to patient satisfaction as well. As results are delayed and the team gets backed up, every hour of delay adds to the patient’s anxiety and fear. Anxiety and fear are not feelings we want our patients to experience, we want them to have confidence in our teams and systems.
Automated applications and integration monitoring can help quickly resolve these problems. With early notifications to the right team in a timely manner, problems can be quickly resolved to minimize downtime impacts. For over 10 years Tido Inc. has been partnering with health systems to help maximize their IT systems and quickly resolve issues as they arise, often before the end user even notices. Contact us today and so we can talk about how we can help you keep your systems working for your clinical teams.